How big data and analytics can strengthen your fraud prevention
With more and more of our lives conducted online – alongside the growth in AI – fraud and cyber threats are only becoming more complex and challenging to deal with. Adopting the latest detection methods are key to staying ahead of the fraud and maintaining trust and security in your ecosystem.
The foundation: data analytics
At its core, fraud detection is a data problem. Each transaction, no matter how innocuous it appears, carries with it a wealth of information – patterns waiting to be deciphered. This is where big data and analytics come into play, transforming seemingly unrelated data points into a coherent narrative that can unveil the hidden story of a potential fraud.
Here is how data analytics is used to prevent fraud effectively:
Pattern recognition: Data analytics can analyse vast amounts of transactional data to identify patterns, trends, and anomalies that may indicate fraudulent activities.
Real-time monitoring: Advanced analytics platforms can provide real-time monitoring of transactions and activities, enabling you to detect and respond to fraudulent behaviour quickly.
Predictive modelling: Data analytics uses predictive modelling techniques to forecast potential fraud risks based on historical data patterns.
Behaviour analysis: Data analytics can review customer behaviour, transaction patterns, and other relevant data to create profiles and identify anomalies that may be indicative of fraud.
Machine learning and AI: By incorporating machine learning and artificial intelligence algorithms into fraud detection systems, you can strengthen your fraud detection and prevention capabilities.
The power of pattern recognition
One of the primary advantages of data analytics lies in its ability to identify and learn from patterns. Analytics excels at pattern recognition, allowing banks to identify subtle anomalies that may go unnoticed by manual reviews. By leveraging machine learning algorithms, deviations from typical customer behaviour - such as sudden high-value transactions in unusual locations - can be flagged for further investigation.
Furthermore, fraud detection algorithms powered by machine learning continuously adapt and refine their predictive accuracy by learning from new data inputs. This evolutionary process leads to more sophisticated systems that can differentiate between genuine anomalies and benign deviations, reducing false positives and making your efforts more efficient.
Opportunities with big data
The advent of big data has revolutionised fraud detection, enabling financial institutions to process and analyse vast datasets in real-time. Techniques like social network analysis can uncover hidden connections between seemingly unrelated accounts, unveiling organised fraud schemes that would have been difficult to detect using traditional methods alone.
Additionally, the integration of artificial intelligence and machine learning technologies into analytics platforms has ushered in a new era of dynamic fraud detection systems. These adaptive systems not only stay ahead of emerging fraud trends but also personalise fraud detection strategies at an individual level, providing a tailored and robust defence against fraudsters.
What to do now
1. Establish a data-driven fraud prevention strategy: Develop a comprehensive fraud prevention strategy that leverages data analytics for pattern recognition, real-time monitoring, predictive modelling, behaviour analysis, and machine learning.
2. Invest in advanced analytics platforms: Consider investing in advanced analytics platforms that offer real-time monitoring capabilities to detect and respond to fraudulent activities swiftly.
3. Use predictive modelling techniques: To forecast potential fraud risks based on historical data patterns. By analysing past fraud incidents and identifying common characteristics, predictive models can help you anticipate and prevent fraudulent activities before they occur.
4. Leverage machine learning and AI: Incorporate these algorithms into your fraud detection systems to enhance detection and prevention capabilities. These technologies can continuously learn from new data inputs, adapt to evolving fraud patterns, and improve the accuracy of fraud management processes.
5. Enhance human expertise: Recognise the importance of your people in fraud prevention and empower your analysts and fraud experts to work with advanced data analytics tools.
Why it matters now
In today's fast-evolving landscape, legacy fraud controls may not be equipped to tackle modern fraud threats effectively. Many fraudulent activities today leave digital traces, offering an opportunity for proactive prevention. Leading banks are using big data and analytics to detect potential fraudulent activities before they materialise. Given the evolving regulatory environment and the persistent challenges of fraud, corruption, and abuse, this can provide you with a critical advantage in navigating this complex terrain.
Related articles
Need expert advice?
Get in touch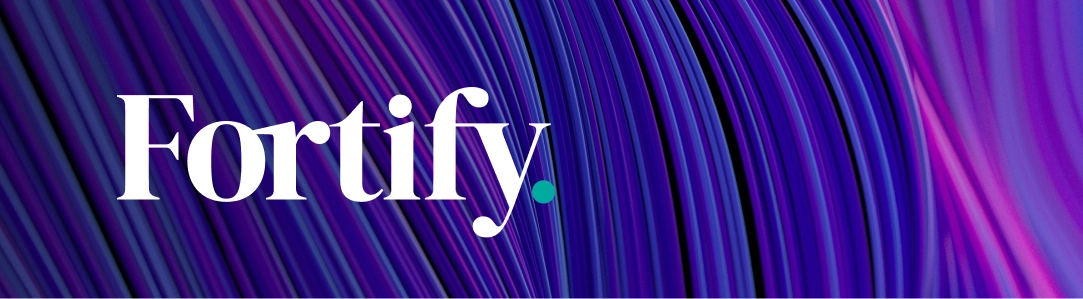
