4 ways to enhance your fraud and risk systems
Financial fraud costs the global economy an estimated US$3.7tn each year, according to Moody’s. For banks, financial crime poses a constant threat despite growing efforts and investment to fight it. Fraudsters can infiltrate even the strongest defences. Let’s explore some key areas where vulnerabilities can arise and what to do.
1. Quality data is key
Imagine training an athlete on a diet of junk food. Fraud systems are no different. They require high-quality data inputs to stay ahead of evolving fraud tactics. Providing your systems with up-to-date and diverse data is essential for their effectiveness.
If a bank simply relies on transaction data from a single source or source type, you may miss out on detecting activities that involve unusual patterns or behaviours that are only evident when data from various sources are analysed together.
By integrating real time data from multiple channels such as ATM transactions, online banking activities, credit card spending, and even geolocation data, the fraud detection system can create a more holistic profile of each customer's typical behaviour. This allows the system to identify anomalies more accurately, such as sudden large transactions from a location the customer has never visited or unusual spending patterns that deviate from the norm.
2. Equip your team with advanced tools
A successful fraud and risk system relies on a skilled team working with the best tools. To succeed, your fraud teams and analysts need robust data analytics, precise model development, and effective monitoring tools.
While advanced tools can be complex to use, they enable a much more sophisticated and accurate detection of fraudulent activities. Machine learning algorithms can analyse large volumes of data in real-time to identify patterns and anomalies.
Many fraud teams use a basic rule-based system to flag potentially fraudulent transactions. In doing that, they can miss out on more subtle forms of fraud that deviate from typical patterns. However, by using advanced machine learning models, the team can detect behaviour that is outside the norm for that customer (and therefore potentially fraudulent).
Moreover, advanced tools like artificial intelligence can continuously learn and adapt to new fraud patterns, allowing the system to evolve and stay ahead of increasingly complex and sophisticated tactics used against banks.
3. Continuously monitor, test, and adjust systems to keep up with evolving threats
It is crucial to remain agile in combating financial crime. Firstly, fraudsters are constantly developing new tactics and techniques to bypass security measures. With continuous monitoring and testing, you can identify areas for improvement, address any vulnerabilities and optimise the performance of your systems. Finally, regulatory requirements are constantly changing, so ongoing monitoring will ensure you remain compliant with industry regulations and best practice.
4. Evaluate your performance using DORA metrics
DORA metrics aren’t just for software; they’re a compass for your analytic systems too. So pinpoint areas of strength and weakness in your fraud systems by asking yourself these questions:
Deployment frequency: Are your system changes fleeting or sluggish?
Deployment frequency measures how often changes or updates are made to the system. A higher deployment frequency can indicate that fraud teams are agile, responsive to new threats, and able to implement improvements quickly to enhance their fraud detection capabilities.
Lead time for changes: From inception to deployment, how swift is the transition?
This metric tracks the time taken from the inception of a change or update to its deployment. A shorter lead time for changes suggests that fraud teams are efficient in implementing new fraud prevention strategies, adapting to emerging threats, and minimising the time between identifying a need for change and implementing it in the system.
Mean time to recovery (MTTR): After an issue, how fast does your system bounce back?
MTTR measures the average time it takes to recover from a system failure or incident. For fraud teams, a low MTTR indicates that you’re able to respond swiftly and effectively to incidents of fraud, minimising their impact.
Change failure rate: How often do your changes not deliver?
This metric evaluates the percentage of changes or updates that result in failure or negative outcomes. A low change failure rate indicates that fraud teams have robust testing procedures in place, implement changes successfully, and avoid introducing vulnerabilities or errors that could compromise the effectiveness of the fraud detection system.
To conclude, enhancing fraud and risk systems is essential in the face of the staggering cost of fraud to banks and consumers. By prioritising quality data inputs, equipping teams with advanced tools, continuously monitoring and adjusting systems, and evaluating performance using DORA metrics, institutions can strengthen their fraud detection capabilities and protect themselves and their customers from financial harm. Embracing these strategies will empower fraud teams to stay ahead of fraudsters, adapt to changing conditions, and effectively combat financial crime in an ever-evolving landscape of risks and threats.
Related articles
Need expert advice?
Get in touch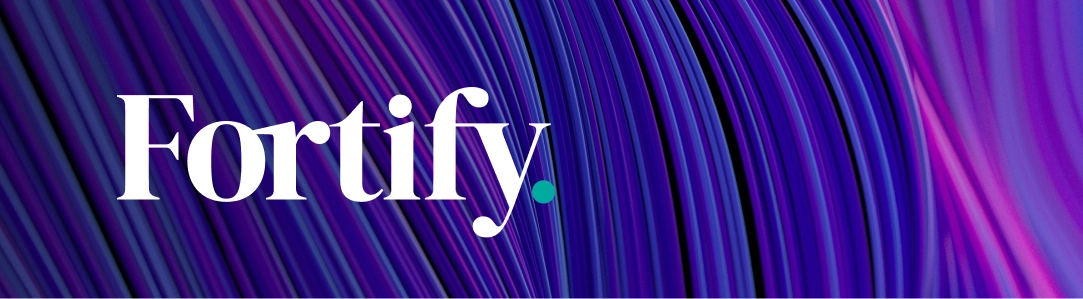
