User Behaviour Analytics in fraud detection: bridging the gap between payments and cybersecurity
In the ever-evolving landscape of fraud prevention, understanding the underlying patterns of user behaviour is crucial. Whether you're in a fraud team at a financial institution or a cybersecurity expert, the principles of User and Entity Behaviour Analytics (UEBA) can provide powerful insights into detecting and preventing unwanted behaviour. Essentially, UEBA uses machine learning to detect anomalies in the behaviour of users and devices connected to a corporate network. At its core, many real-time decision systems in both fields are responding to events driven by human behaviour. Let’s understand how we apply it to payment fraud detection.
The intersection of payments and cybersecurity: UEBA’s role
These ideas were originally developed for an audience in the Payments Village at DEFCON which attracts experts from a range of diverse industries from banking to cybersecurity to eCommerce. I’m always keen to learn as much as I can from other sectors, and in return this talk offers some new ways for them to think about their problems. I lift the lid on how we use behavioural analytics to prevent fraud and draw parallels with strategies used cybersecurity.
Cybersecurity teams are familiar with UEBA as it applies to network security. But many of the same principles can be applied to payment fraud detection. At first glance, payment messages that fraud teams analyse may seem far removed from network logs in cybersecurity, but they share more similarities than you might think.
A payment message, at its heart, is a log containing four key fields: sender, receiver, time, and amount. Just like network logs, these messages are transported between various systems, with additional details layered on top – such as merchant addresses, postcodes, and security checks. By analysing these logs using a user behaviour lens, we can uncover patterns and anomalies that may indicate fraudulent activity.
The growing threat of social engineering in payments
One of the most significant challenges in payments today is social engineering, often referred to as scams or authorised payment fraud. Defined by Interpol as ‘scams used by criminals to exploit a person's trust in order to obtain money directly or obtain confidential information to enable a subsequent crime’. The scale of this issue is staggering – in 2022, Americans lost over $8 billion to social engineering scams. While losses reached $600 million in theUK and $1.8 billion in Australia. The growth of this type of scam is largely due to the increased difficulty of stealing money through weak banking application security. As a result, scammers have turned to targeting people via sophisticated methods like romance scams, investment fraud, and even deep-fake CEOs.
What’s also concerning for those in the finance industry is the trend towards making banks liable for these losses. In the UK, a deadline of 5 October 2024 has been set for when banks will be held accountable, and similar discussions are happening in the US regarding Regulation E.
The data footprint of scams
When it comes to detecting this type of scam, fraud teams are often limited in the data they have access to. While scams may unfold through a Facebook ad or a phone call ‘from the bank,’ the data available to fraud teams is typically restricted to payment messages. However, even with this limited data, it’s possible to identify suspicious accounts that are being used to receive stolen money and launder it through other accounts.
A common pattern is that these accounts will see a large influx of money, only for the same amount to be transferred out within a few days to different accounts. By focusing on these behaviours, fraud teams can begin to identify and prevent fraudulent activity before it escalates.
A typical example might look like the sequence of transactions in the table below.

And yet, even this seeming ‘slam dunk’ example of a scam might prove to be something else depending on the context – that is the all-important history of both the sending and receiving accounts.
Quantifying suspicion: using behavioural analytics to understand historical behaviour
The ultimate aim of UEBA in fraud detection is to quantify suspicion in a way that is highly predictive of fraud. This involves creating metrics that generalise across various scenarios and accurately capture what is suspicious about a particular pattern of behaviour.
One of the main methods for achieving this is by aggregating ‘historical behaviour’ for the accounts involved in a transaction. For example, if an account suddenly processes $20,000 in a day, this could be flagged as suspicious.
Would you be more or less suspicious of the transaction sequence starting 2024-08-01 given the prior transactions on the account?

When analysing suspicious behaviour, domain knowledge is very helpful. For example, a dormant account suddenly receiving large sums of money, or a new account being used to transfer funds in and out, are both red flags. It’s also important to consider the timing of these transactions – was there a significant spike in activity on a particular day?
But beyond these surface-level indicators, it’s crucial to measure not just the raw amount of money, but also other factors like the percentage of the account balance that is moving. This helps to further pinpoint unusual behaviour and truly identify outliers.
What is trust? A quantifiable metric in fraud detection
Trust is another critical factor in UEBA, particularly in the context of payments. For our purposes, trust is defined as a long history of good behaviour. Scammers often try to game the system by gaining access to established accounts, typically through schemes like ‘work from home’ jobs.This type of scam sees fraudsters create fake job postings and then steal your personal information or financial assets. So, by analysing the history of an account and looking for consistent behaviour, fraud teams can better assess the risk associated with a particular transaction.
Let’s look at the same sequence of transactions from earlier but with some of the money senders and receivers highlighted with names.

Now with some additional context, how suspicious does this look? Can you think of a way to quantify your intuition?
Putting all the signals together
In my experience with UEBA systems, one more complex metric that combines several indicators of risk together provides much more effective risk signals than simper systems.
Here I suggest one possibility of what such a complex risk metric could look like when we put several risk measures together; trust, account activity history, total $. I dub this metric ‘suspicious percentage flow’ for the account. This is computed as a percentage of the prior day’s account balance that is moving in the current transaction, weighted by the suspicion score of the receiver account. That was definitely a mouthful, but the table illustrates the computation of the metric.

Can you spot the fraud? Is there a better way you can think of to combine these indicators into an even sharper metric that identifies risk? Please get in touch and let us know!
Conclusion: sharpening the definition of risk in payments
In summary, applying UEBA to payments is all about measuring the riskiness of a transaction by aggregating risky activities associated with an account. This requires continuous refinement and testing of risk measures, along with a deep understanding of the domain. The dispersion of genuine activity is vast, so it’s essential to sharpen our measures of risk by incorporating both activity and trust metrics.
While we’ve explored just one possible risk measure in this discussion, it’s important to remember that typical scam detection models often rely on 100 or more risk measures of similar complexity. By integrating these insights into our fraud detection strategies, we can better protect our financial systems and reduce the impact of fraud on consumers and businesses alike. And for other industries in the room, like cybersecurity, these ideas offer new ways for them to become more targeted in the interventions their systems make. The challenge as always is to find tools that not only enable this type of experimentation but make it possible to deploy these detections seamlessly to production. Something that Fortify will be working on in the future so watch this space!
Related articles
Need expert advice?
Get in touch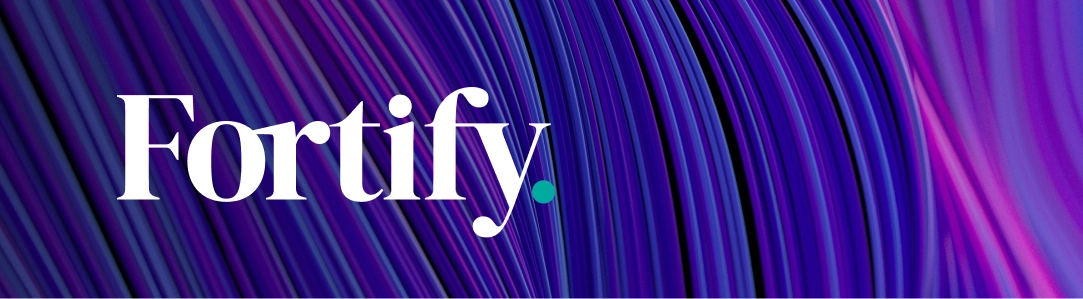
